In an age of IoT, smartphones, and dozens upon dozens of connected devices in our home, there is more data than ever for marketers to use and make informed decisions about customers, right?
In fact, I get many calls from a lot of data companies that can’t make sense (meaning, making valuable) of all the data there ingesting. The old saying goes, “water, water everywhere but not a drop to drink”.
Data-driven decision-making is not a bad idea but when the wrong data is built into that methodology or system, it’s not going to work well. The best case, it’s reactive. Knowing when customers are going to be loyal after you see a bunch of people visiting the shop or coming to our website over and over again is not a prediction. Some people might value it but they’re going to value it low. That’s not a scalable businH
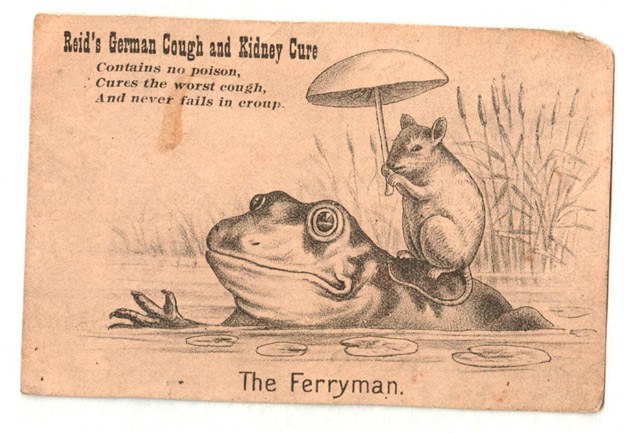
The problem as I see it, people are complicated. Even though we have a lot of data about people’s actions, we’re not really looking at the root cause reasons why they make decisions. We’re looking at evidence of past decision-making and in many cases, it’s just a bunch of data that’s correlating to nothing valuable.
What’s more important than the data is how you think about data. Executives are often relied upon to make decisions and to use what’s in front of them. Most always they don’t have enough of the right information to make non-bias, good decisions that are predictive in nature. I was once told by an executive who said I wait for perfect information before I make a decision. Wow.
Two things can get you out of this mess. One, create data where there was none. Be predictive. That is, create information that is causal in nature and is a hypothesis about the future. There are ways to do it. Two, learn to be less wrong. What I mean by that is there are ways to be less wrong than to try to be right all the time. It does not sound like a great idea but how are you going to manage risk when there are more unknowns than you can ever quantify? If we think events are certain, there’s a way to manage that and plan for it but chances are there’s a lot of uncertainty in what you’re attempting to do. We think it’s uncertain times now, just realize that over 90% of all products fail – every decade for the last 30+ years. Imperfect data has always been the case and it’s not any better even if we have all of this so-called information.
The concept of being less wrong comes from Thomas Bayes who was an English mathematician and in 1763 came up with the idea of Bayesian mathematics. It’s a simple idea with profound mathematics behind it. When we accumulate more data with unproven evidence how can we predict the probability of an event if you only have partial information?
It turns out you can collect a lot of partial data points. The right ‘math glue’, you can determine where not to go. For business leaders, it’s kind of hard to imagine that 98+% of all those people out there will never be your customer. You can construct the model to prove why (its sometimes best to ignore this and market to 98% of the population) If we narrow down the area of focus, this increases the probability of success for what remains. This allows you to not necessarily proven outcome but removes the noise. Over time you will incorporate new data, further increasing the likelihood of success – Call it systematic intelligence. Now you were approaching evidence of causality because you can truly predict outcome. As less wrong keeps getting better soon becomes prediction.
Alan Turing use this thinking to develop the machines necessary to break the German codes during World War II. Also, during World War II this method was used well to predict German military production which allowed planners in the United States to allocate resources for more accurately. Fast forward to today, the same thing holds true.
Now I had artificial intelligence and you have a system that can truly move fast. Just don’t give it a bunch of junk. In a wanabee deterministic world, good decision making under duress, in a complex and forever-changing world requires a better approach.